Machine Learning for Automated Detection of Shipwreck Sites from Large Area Robotic Surveys
May 23 - June 3, 2022 / June 5 - 23, 2023
Expedition Overview
From June 5 - 23, 2023, an expedition team will perform field trials to test and validate new methodologies for the use of autonomous underwater vehicles (AUVs) to search for and survey shipwreck sites in Lake Huron’s Thunder Bay National Marine Sanctuary.
These field trials are a continuation of work that took place from May 23 - June 3, 2022, during which the team worked on NOAA Great Lakes Environmental Research Laboratory’s Research Vessel Storm to deploy autonomous underwater vehicles (AUVs) and remotely operated vehicles (ROVs). These systems collected sonar data in areas with a high density of known shipwreck sites. The data collected were then used to train and validate the team’s machine learning models with novel algorithms developed for shipwreck detection from sonar imagery. With their ROV and AUV platforms equipped with cameras for recording images and videos of underwater sites, the expedition team also assessed the conditions of these targeted regions to guide future field testing.
Building on knowledge and data gathered in the previous year, the team will focus on field trials during their 2023 expedition. The team’s main focus will be to field test and validate each individual component of their model — including new machine learning algorithms for the detection and ranking of potential shipwreck sites and new methods to efficiently plan robotic surveys — as well as their full pipeline for the autonomous search and survey of shipwreck sites. The team will also use these systems to perform exploratory searches for shipwrecks in areas that have been identified as having high potential for new discovery.
Due to its maritime history and strategic location, Thunder Bay National Marine Sanctuary contains almost 100 known shipwreck sites and over 100 shipwrecks left to be found, thus offering a promising potential for both shipwreck discovery and development of machine learning algorithms. Shipwrecks help us better understand our past. But discovering and exploring them is expensive, time-consuming, and labor intensive. By advancing and training the capabilities of marine robotic systems to search for and survey shipwreck sites autonomously, the expedition team aims to increase the efficiency and decrease the costs associated with such exploration efforts.
Data collected and software developed throughout this project will not only inform and enhance public education and the management and conservation of important sanctuary resources, but also be widely applicable to the discovery of submerged maritime assets in the deep ocean. The data and software will be made publicly available and will serve as a benchmark for future research at the intersection of machine learning and ocean exploration.
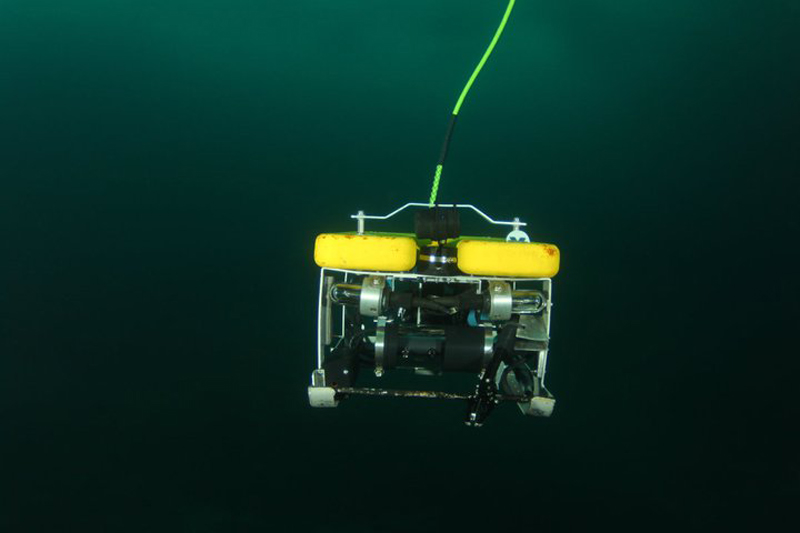
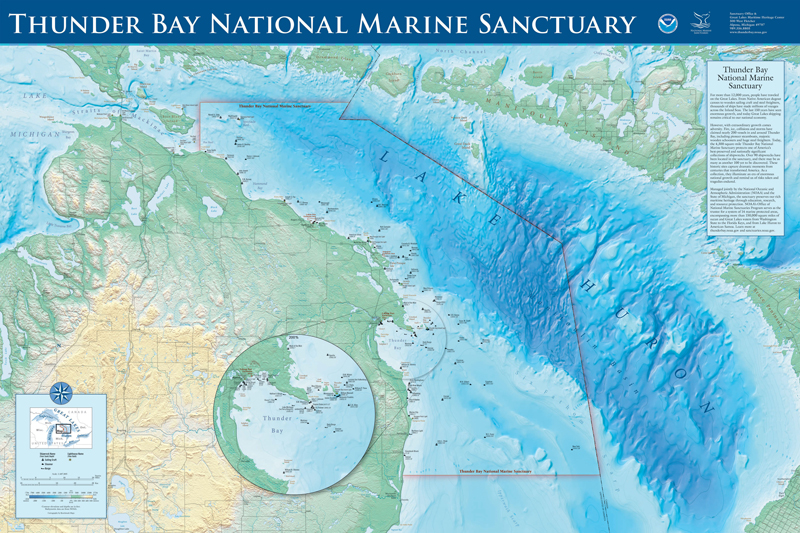